Surface Duo Blog
Build great Android experiences, from AI to foldable and large-screens.
Latest posts
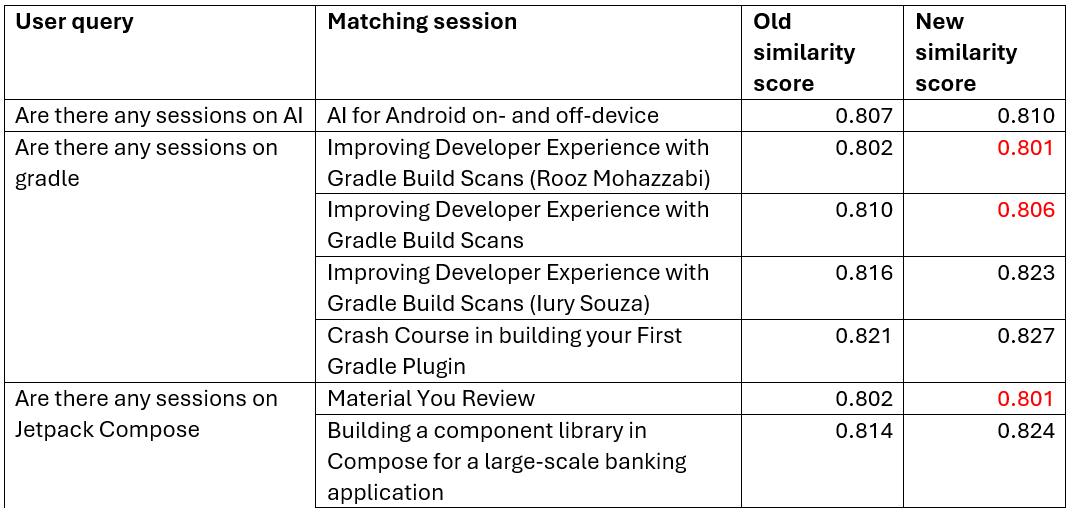
More efficient embeddings
Hello prompt engineers, I’ve been reading about how to improve the process of reasoning over long documents by optimizing the chunking process (how to break up the text into pieces) and then summarizing before creating embeddings to achieve better responses. In this blog post we’ll try to apply that philosophy to the Jetchat demo’s conference chat, hopefully achieving better chat responses and maybe saving a few cents as well. Basic RAG embedding When we first wrote about building a Retrieval Augmented Generation (RAG) chat feature, we created a ‘chunk’ of information for each conference session....
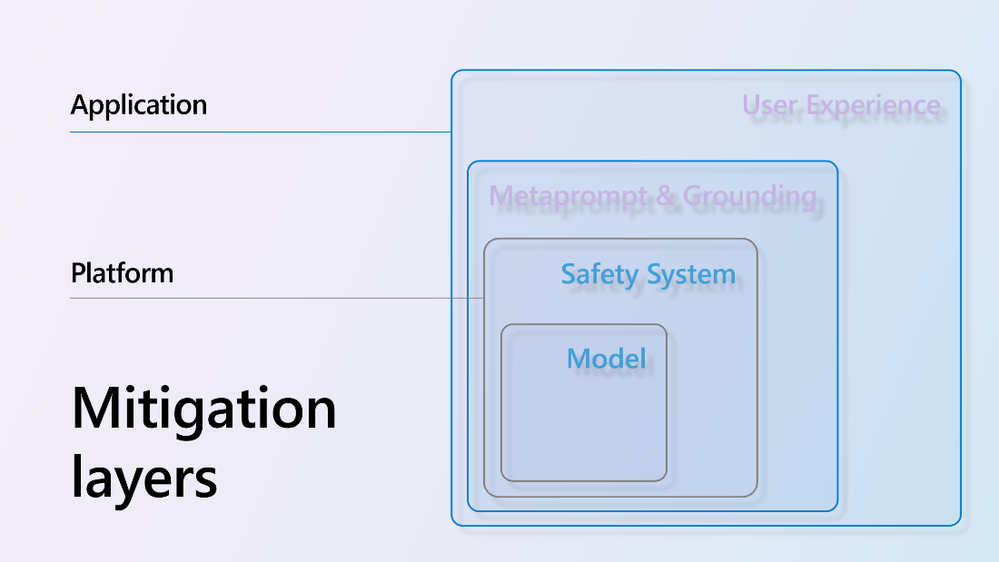
Responsible AI and content safety
Hello prompt engineers, This week we’re taking a break from code samples to highlight the general availability of Azure AI Content Safety. In this blog series we’ve touched briefly on the using prompt engineering to restrict the types of responses an LLM will provide, such as setting the system prompt to set boundaries on what questions will be answered: Figure 1: System prompt set to "You will answer questions about the speakers and sessions at the droidcon SF conference." However, ensuring a high-quality user experience goes beyond simple guardrails like this. You want your application’...

“Search the web” for up-to-date OpenAI chat responses
Hello prompt engineers, Over the course of this blog series, we have investigated different ways of augmenting the information available to an LLM when answering user queries, such as: However, there is still a challenge getting the model to answer with up-to-date “general information” (for example, if the question relates to events that have occurred after the model’s training). You can see a “real life” example of this when you use Bing Chat versus ChatGPT to search for a new TV show called “Poker Face” which first appeared in 2023: Figure 1: ChatGPT 3.5 training end...
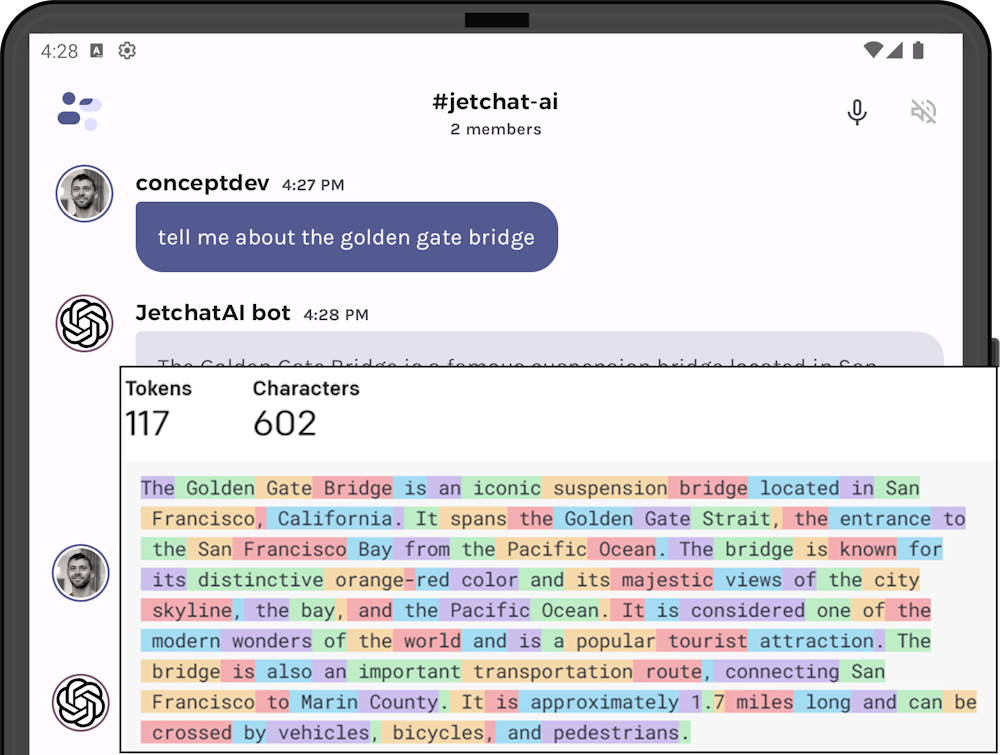
Android tokenizer for OpenAI
Hello prompt engineers, The past few weeks we’ve been extending JetchatAI’s sliding window which manages the size of the chat API calls to stay under the model’s token limit. The code we’ve written so far has used a VERY rough estimate for determining the number of tokens being used in our LLM requests: This very simple approximation is used to calculate prompt sizes to support the sliding window and history summarization functions. Because it’s not an accurate result, it’s either inefficient or risks still exceeding the prompt token limit. Turns out that there is an Android-compatible ...
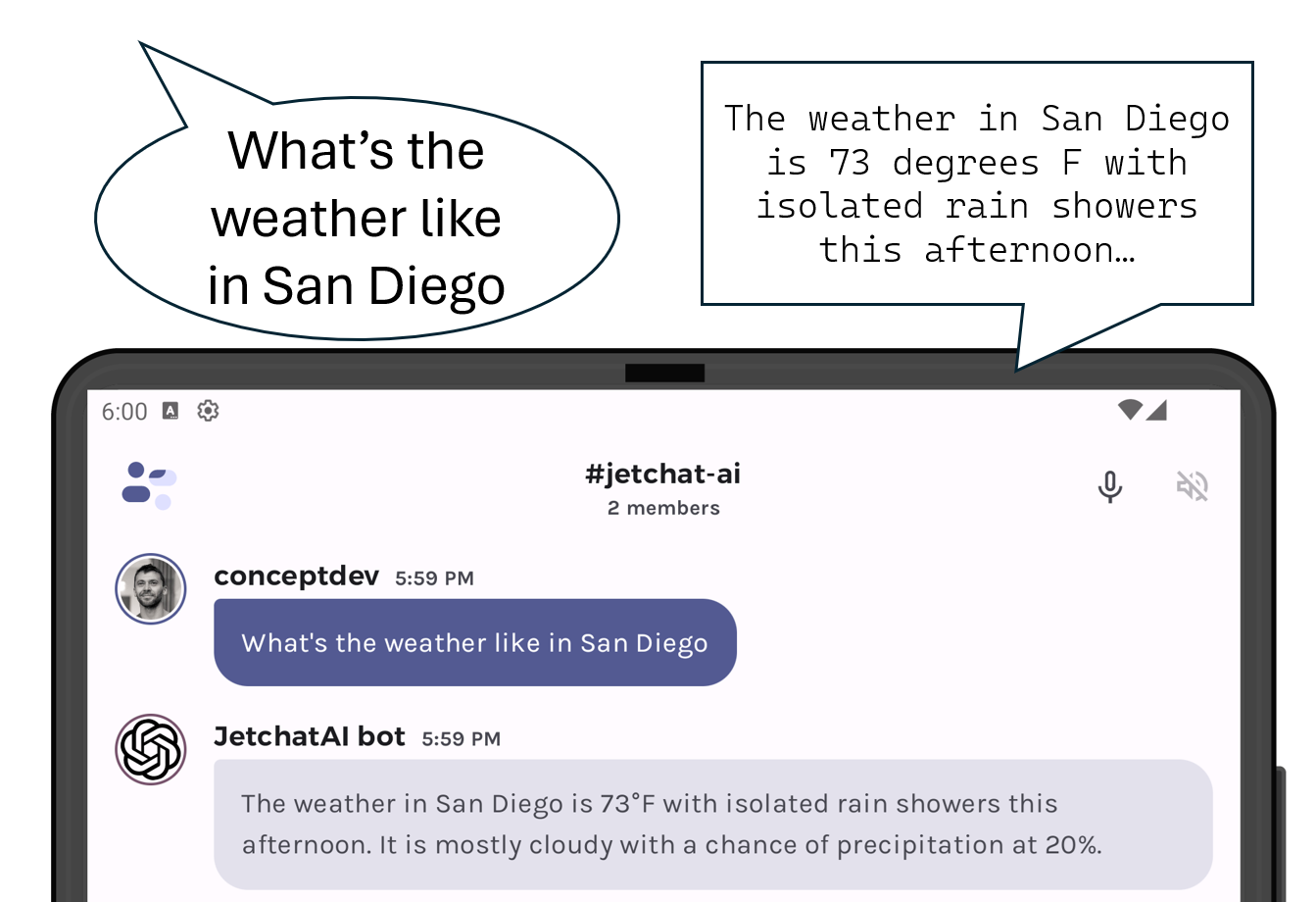
Speech-to-speech conversing with OpenAI on Android

Hello prompt engineers, Just this week, OpenAI announced that their chat app and website can now ‘hear and speak’. In a huge coincidence (originally inspired by this Azure OpenAI speech to speech doc), we’ve added similar functionality to our Jetpack Compose LLM chat sample based on Jetchat. The screenshot below shows the two new buttons that enable this feature: Figure 1: The microphone and speaker-mute icons added to Jetchat The speech that is transcribed will be added to the chat as though it was typed and sent directly to the LLM. The LLM’s response is then automati...
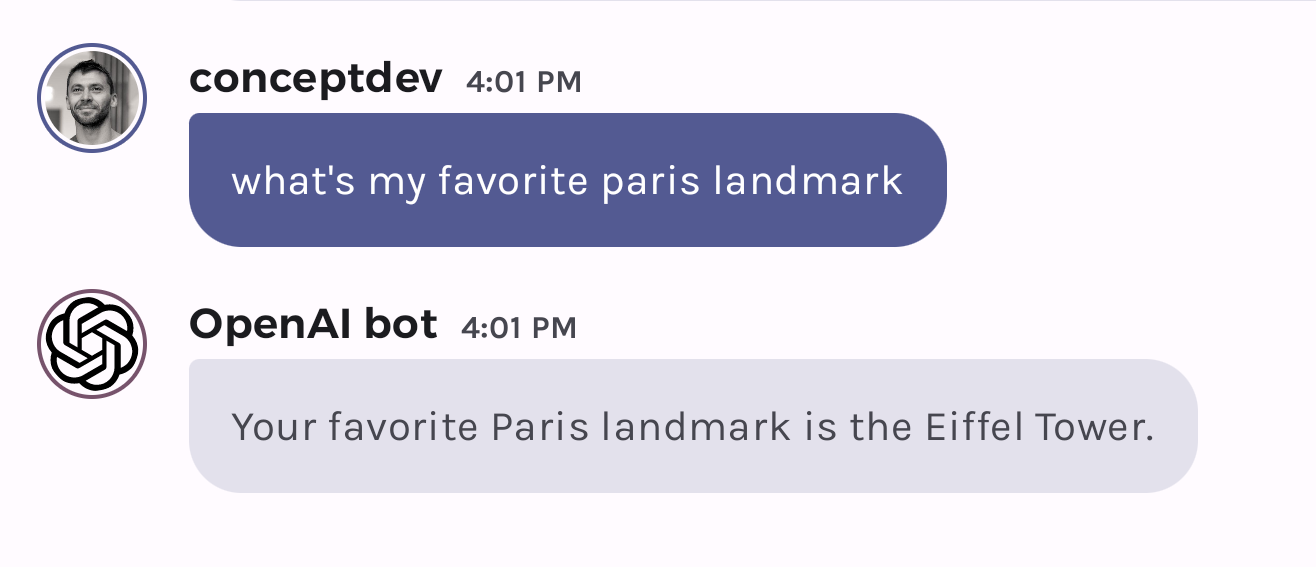
Infinite chat with history embeddings
Hello prompt engineers, The last few posts have been about the different ways to create an ‘infinite chat’, where the conversation between the user and an LLM model is not limited by the token size limit and as much historical context as possible can be used to answer future queries. We previously covered: These are techniques to help better manage the message history, but they don’t really provide for “infinite” memory. This week, we will investigate storing the entire chat history with embeddings, which should get us closer to the idea of “infinite chat”. One of the first fe...
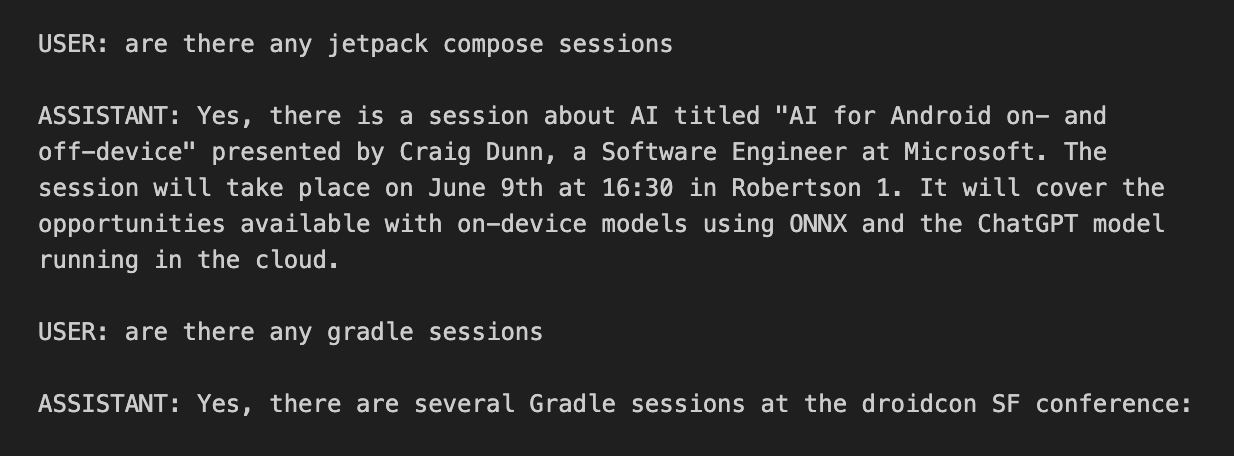
“Infinite” chat with history summarization
Hello prompt engineers, A few weeks ago we talked about token limits on LLM chat APIs and how this prevents an infinite amount of history being remembered as context. A sliding window can limit the overall context size, and making the sliding window more efficient can help maximize the amount of context sent with each new chat query. However, to include MORE relevant context from a chat history, different approaches are required, such as history summarization or using embeddings of past context. In this post, we’ll consider how summarizing the conversation history that’s beyond the slidi...
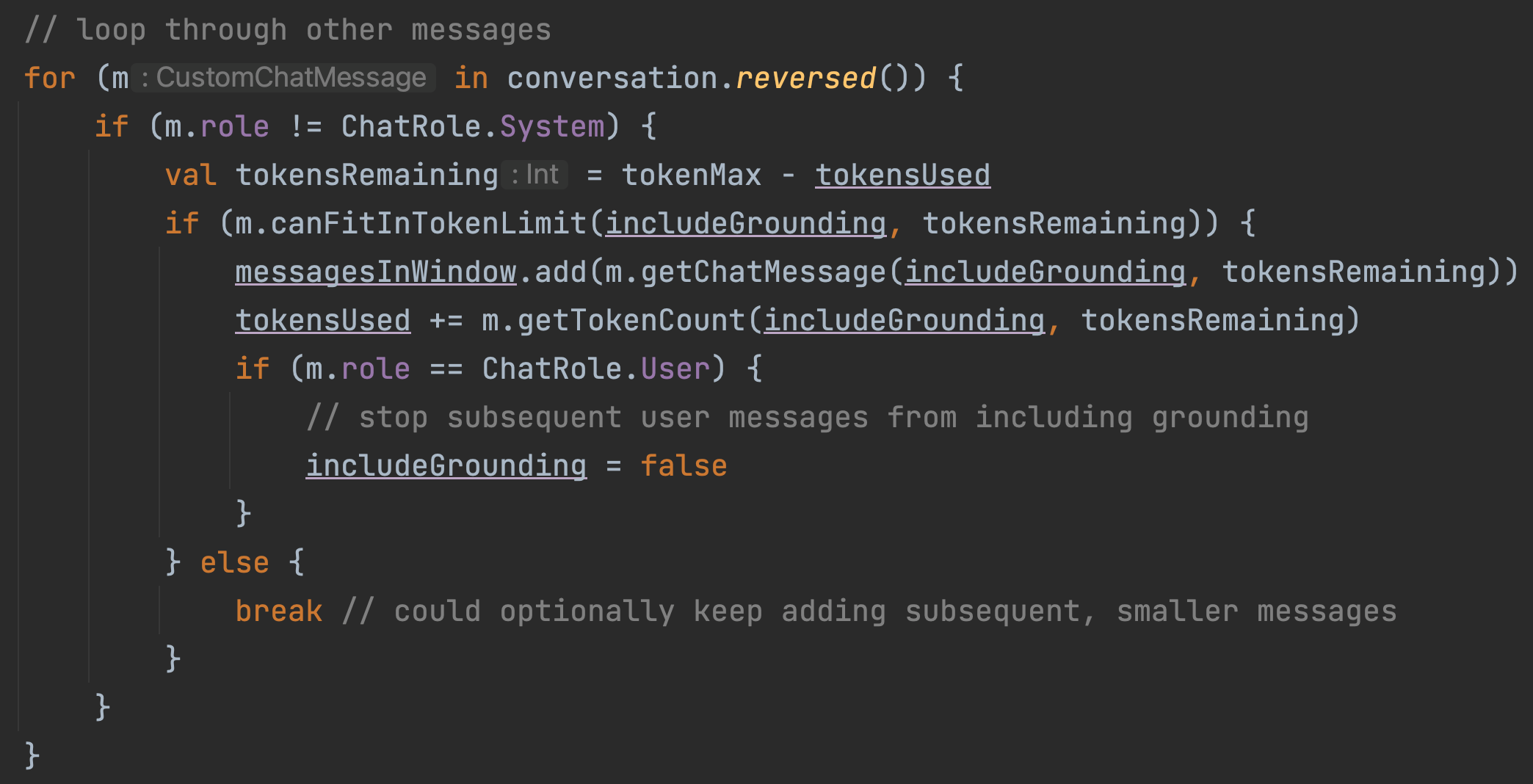
De-duplicating context in the chat sliding window
Hello prompt engineers, Last week’s post discussed the concept of a sliding window to keep recent context while preventing LLM chat prompts from exceeding the model’s token limit. The approach involved adding context to the prompt until we've reached the maximum number of tokens the model can accept, then ignoring any remaining older messages and context. This approach doesn’t take into account that some context is duplicated when the results are augmented with embeddings or local functions, because the request contains the augmented source data AND the model’s response contains the relevant in...
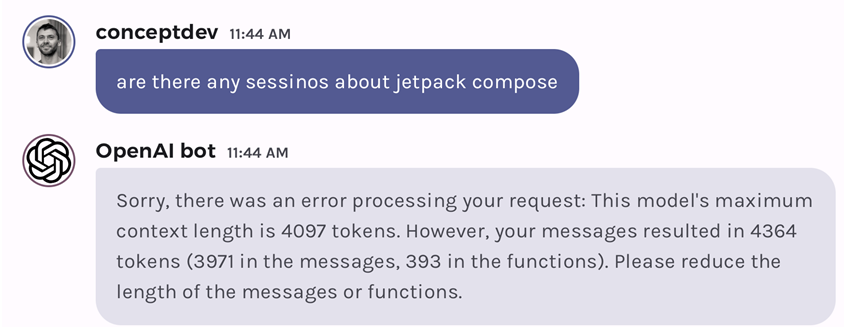
Infinite chat using a sliding window
Hello prompt engineers, There are a number of different strategies to support an ‘infinite chat’ using an LLM, required because large language models do not store ‘state’ across API requests and there is a limit to how large a single request can be. In this OpenAI community question on token limit differences in API vs Chat, user damc4 outlines three well-known methods to implement infinite chat: The thread also suggests tools like Langchain can help to implement these approaches, but for learning purposes, we’ll examine them from first principles within the context of the...